Nari Labs' Dia-1.6B Outperforms Competitors in Emotional Speech
Nari Labs has introduced Dia-1.6B, an open-source text-to-speech model that claims to surpass established competitors like ElevenLabs and Sesame in generating emotionally expressive speech. Despite its small size, with just 1.6 billion parameters, Dia-1.6B can create realistic dialogue complete with laughter, coughs, and emotional inflections, including the ability to scream in terror. This model runs in real-time on a single GPU with 10GB of VRAM, processing about 40 tokens per second on an Nvidia A4000. It is freely available under the Apache 2.0 license through Hugging Face and GitHub repositories.
While other AI models can simulate screaming, Dia-1.6B stands out by understanding the context in which a scream is appropriate, making it a more natural and organic response. This capability is highlighted by the fact that even advanced models like OpenAI’s ChatGPT struggle with conveying such nuanced emotions. Nari Labs co-founder Toby Kim emphasized the model's ability to handle standard dialogue and nonverbal expressions better than competitors, which often flatten delivery or skip nonverbal tags entirely.
The development of emotionally expressive AI speech remains a significant challenge due to the complexity of human emotions and technical limitations. The "uncanny valley" effect, where synthetic speech sounds human but fails to convey nuanced emotions, is a persistent issue. Researchers are employing various techniques to address this, including training models on datasets with emotional labels and using deep neural networks to analyze contextual cues. However, the technology is still far from convincing, and most models tend to create an uncanny valley effect that diminishes user experience.
ElevenLabs, a market leader, interprets emotional context directly from text input, looking at linguistic cues, sentence structure, and punctuation to infer the appropriate emotional tone. Its flagship model, Eleven Multilingual v2, is known for its rich emotional expression across 29 languages. OpenAI recently launched "gpt-4o-mini-tts" with customizable emotional expression, highlighting the ability to specify emotions like "apologetic" for customer support scenarios. However, its Advanced Voice mode is so exaggerated and enthusiastic that it could not compete in tests against other alternatives like Hume.
Dia-1.6B potentially breaks new ground in handling nonverbal communications. The model can synthesize laughter, coughing, and throat clearing when triggered by specific text cues, adding a layer of realism often missing in standard TTS outputs. Beyond Dia-1.6B, other notable open-source projects include EmotiVoice—a multi-voice TTS engine that supports emotion as a controllable style factor—and Orpheus, known for ultra-low latency and lifelike emotional expression.
The challenge of emotional speech synthesis lies in the lack of emotional granularity in training datasets. Most datasets capture speech that is clean and intelligible but not deeply expressive. Emotion is not just tone or volume; it is context, pacing, tension, and hesitation. These features are often implicit and rarely labeled in a way machines can learn from. Even when emotion tags are used, they tend to flatten the complexity of real human affect into broad categories like 'happy' or 'angry,' which is far from how emotion actually works in speech.
AI systems often perform poorly when tested on speakers not included in their training data, an issue known as low classification accuracy in speaker-independent experiments. Real-time processing of emotional speech requires substantial computational power, limiting deployment on consumer devices. Data quality and bias also present significant obstacles, as training AI for emotional speech requires large, diverse datasets capturing emotions across demographics, languages, and contexts. Systems trained on specific groups may underperform with others, and some researchers argue that AI cannot truly mimic human emotion due to its lack of consciousness.
Despite these challenges, Dia-1.6B represents a significant step forward in the development of emotionally expressive AI speech. Its ability to understand context and convey nuanced emotions makes it a valuable tool for human-machine interaction. However, the technology is still far from perfect, and further research is needed to overcome the technical hurdles and create more convincing emotional AI speech.
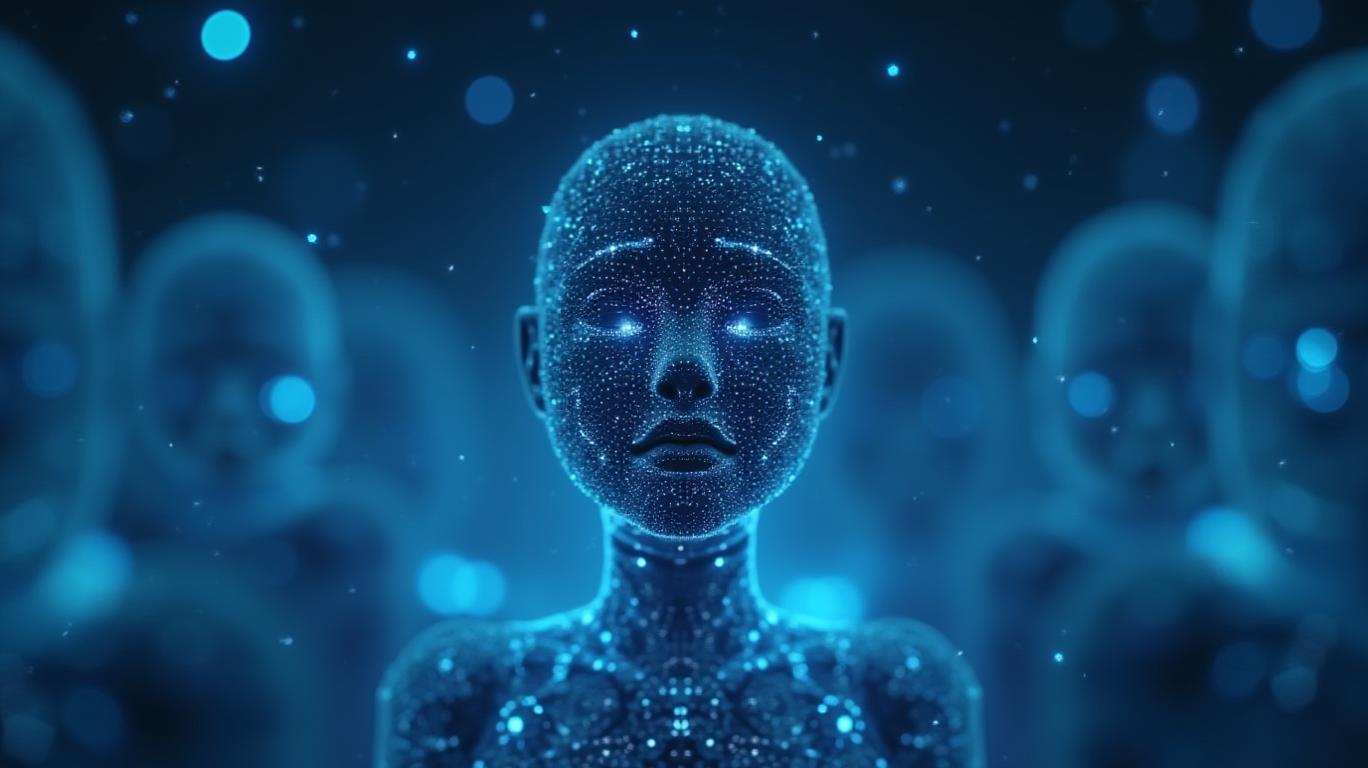