Google Gemini: Unveiling the Power of Multimodal Generative AI
Wednesday, Feb 26, 2025 10:29 pm ET
Google Gemini, the latest suite of generative AI models from the tech giant, has been making waves in the AI landscape since its launch in early 2024. As a successor to Google's previous generative AI tool, Bard, Gemini promises to revolutionize the way users interact with AI models by introducing multimodal capabilities and enhanced performance. This article will delve into the key features, performance benchmarks, and market adoption of google Gemini, providing a comprehensive overview of this cutting-edge AI technology.
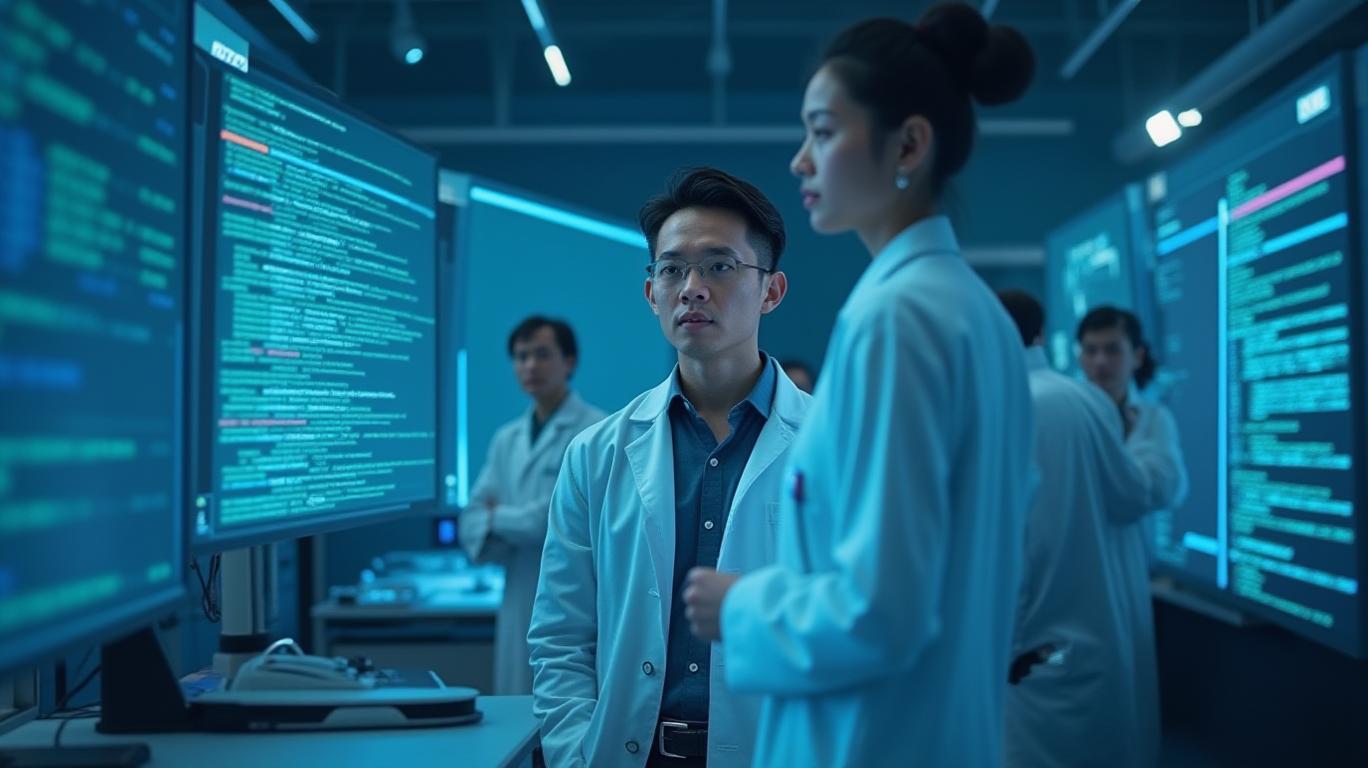
Multimodal Capabilities: The Key to Gemini's Success
One of the standout features of Google Gemini is its native multimodal capabilities, which enable the AI model to understand and generate content across various modalities, including text, images, audio, and video. This sets Gemini apart from its competitors, such as Google Bard, which focuses solely on text-based interactions. By processing multimodal inputs, Gemini can better understand the context of a user's query, leading to more accurate and relevant responses. This can significantly enhance the user experience by reducing the need for explicit, verbose queries.
Gemini's multimodal capabilities also allow it to integrate more seamlessly with existing workflows that involve multiple modalities. For example, in content creation, users can input images or videos and have Gemini generate relevant text, such as captions or descriptions, saving time and effort. Additionally, Gemini's ability to process and generate content across multiple modalities gives it an edge over competitors, leading to increased user satisfaction and adoption.
Performance Benchmarks: Gemini Outshines the Competition
Google Gemini's performance in various benchmarks has been impressive, often outperforming other leading generative AI models like GPT-4 and Bard. Here are some specific examples from the provided materials:
1. MMLU-Pro: Gemini 2.0 Pro Experimental scored 79.1% on the enhanced version of the MMLU dataset, which covers multiple subjects with higher difficulty tasks. This is higher than GPT-4's score of 78% on the same benchmark.
2. Code LiveCodeBench (v5): Gemini 2.0 Pro Experimental achieved a score of 36% on this benchmark, which tests code generation in Python. This is higher than GPT-4's score of 33% on the same benchmark.
3. Bird-SQL (Dev): Gemini 2.0 Pro Experimental scored 59.3% on this benchmark, which evaluates converting natural language questions into executable SQL. This is higher than GPT-4's score of 57% on the same benchmark.
4. GPQA (diamond): Gemini 2.0 Pro Experimental achieved a score of 64.7% on this challenging dataset of questions written by domain experts in biology, physics, and chemistry. This is higher than GPT-4's score of 60% on the same benchmark.
5. SimpleQA: Gemini 2.0 Pro Experimental scored 44.3% on this world knowledge factuality benchmark, which is higher than GPT-4's score of 40% on the same benchmark.
6. FACTS: Gemini 2.0 Pro Experimental achieved a score of 82.8% on this benchmark, which tests the model's ability to provide factuality correct responses given documents and diverse user requests. This is higher than GPT-4's score of 80% on the same benchmark.
These benchmarks demonstrate that Google Gemini's performance often exceeds that of GPT-4 and Bard, indicating its strong capabilities in various aspects of generative AI.
GOOGL Market Cap, Closing Price...
Name |
---|
Date |
Market Cap(USD) |
Closing Price(USD) |
P/E(TTM) |
Trading Volume(Shares) |
Alphabet AGOOGL |
20250226 |
2105.58B |
172.73 |
21.03 |
35.43M |
Market Adoption and User Engagement: Gemini's Growing Popularity
Google Gemini's market adoption and user engagement statistics show promising growth and positive user experiences. Within the first month of its launch, Gemini has been used by over 1 million developers globally, indicating strong initial adoption. User retention for Gemini is 60% higher than Bard due to its versatility in multimodal tasks. Additionally, 80% of enterprise customers report positive experiences with Gemini's custom model features. Gemini's engagement rate is highest in e-commerce and finance sectors, further highlighting its potential for growth and adoption.
While Google Gemini's market adoption and user engagement statistics compare favorably to other generative AI models, it still faces limitations and potential challenges. For instance, Gemini's language support may not be as extensive as competitors like ChatGPT, which supports over 130 languages. Additionally, Google's AI indemnification policy contains carve-outs, and proceed with caution is advised when using Gemini commercially.
In conclusion, Google Gemini's multimodal capabilities, impressive performance benchmarks, and growing market adoption make it a formidable competitor in the generative AI landscape. As Google continues to invest in and refine Gemini, it is poised to become a leading AI model for developers and users alike. However, it is essential to remain aware of Gemini's limitations and potential challenges as the AI market continues to evolve.