AI Agents Revolutionize Web3, Overcoming Computational Challenges
AI agents are revolutionizing the Web3 landscape by autonomously scanning markets, optimizing yields, and securing protocols across various chains. However, the increasing demand for computational resources is posing significant challenges. While discussions on social media platforms highlight the potential of AI agents in decentralized finance (DeFi) and governance, engineers are grappling with the reality that running these agents at scale requires unprecedented computational power. A few projects have successfully addressed this infrastructure challenge, developing systems that make AI agents viable in Web3.
Understanding this infrastructure evolution is crucial for anyone involved in building or investing in decentralized systems. The next wave of Web3 innovation depends on getting these foundational elements right. AI agents in Web3 are not typical automation tools; they are specialized programs designed to handle unique constraints and complexities. Every decision made by these agents involves calculating gas costs across multiple chains, verifying trades through zero-knowledge proofs, and maintaining decentralization without the ability to spin up more cloud servers during demand spikes.
For instance, a DeFi trading bot must juggle cross-chain liquidity pools, predict gas wars, and make split-second decisions while maintaining decentralization. The computational demands escalate when considering real-time market data from multiple chains, smart contract interactions, and cryptographic proofs for every transaction. This complexity explains why early Web3 AI agents failed, either compromising on decentralization or choking on processing demands. The breakthrough came when teams built new systems from the ground up, specifically designed for Web3's unique challenges.
Several projects have moved beyond theoretical discussions and deployed working solutions showcasing the practical potential of AI agents. In the DeFi space, one platform leverages AI agents to monitor market conditions across multiple chains simultaneously. When volatility increases, these agents calculate optimal lending strategies and adjust collateral requirements faster than any human trader. The system processes massive amounts of cross-chain data to predict market movements, often spotting opportunities hours before they become obvious to manual traders.
Another crucial application is in the verification space, where a system combines zero-knowledge proofs with AI inference engines. Each decision made by these AI agents generates a cryptographic proof, creating an auditable trail without sacrificing computational efficiency. This breakthrough enables the confident deployment of AI-driven decisions while maintaining security standards in DeFi protocols.
The computational demands of running AI agents at scale exposed a critical weakness in Web3's infrastructure. One solution emerged from the observation that massive amounts of computing power sit idle in personal devices around the world. By building decentralized peer-to-peer infrastructure networks (DePIN) to harness this untapped resource, a new infrastructure layer was created. This layer reduces operational costs significantly compared to centralized providers and grows organically as demand increases, with new nodes joining the network to provide additional computing power.
This distributed setup tackled core problems head-on. When an AI agent needs to process complex calculations across multiple chains, the workload spreads across the network rather than overwhelming a single node. The architecture ensures consistent performance globally while maintaining the censorship resistance that makes Web3 valuable. Across Web3, teams feel the impact of these infrastructure upgrades, allowing AI agents to handle more sophisticated tasks without compromising on decentralization principles.
The recent success of projects stems directly from these infrastructure advances. Their AI agents operate effectively because the underlying computational framework finally matches their requirements. This shift from theoretical possibility to practical implementation represents years of work solving fundamental technical challenges.
Web3's infrastructure evolution creates unique possibilities for AI agent deployment. Early experiments focus on obvious use cases such as trading, security monitoring, and yield optimization. However, the real transformation lies in unexplored territory. For instance, with decentralized identity systems, AI agents could analyze cross-chain reputation data while preserving privacy through zero-knowledge proofs. Projects are developing frameworks where these agents help assess creditworthiness without accessing sensitive personal information. The computational demands for this kind of analysis would have made it impossible even a year ago.
Community governance represents another frontier. AI agents are beginning to assist decentralized autonomous organizations (DAOs) with treasury management and proposal analysis. For example, when a dao considers a complex DeFi strategy, AI agents simulate potential outcomes across multiple market scenarios. The results help token holders make more informed decisions while maintaining decentralized control.
The success of AI agents in Web3 hinges on more than raw computing power. Infrastructure providers face ongoing challenges around data availability, cross-chain communication, and scalability. Solutions require a careful balance between automation efficiency and decentralization principles. Theoretical models shatter when they hit mainnet, as market volatility, network congestion, and evolving security threats create edge cases that even sophisticated AI agents struggle to handle. Each failure provides valuable lessons, pushing development toward more robust solutions.
The next phase of development focuses on standardization and interoperability. As more projects deploy AI agents, the need for common frameworks becomes clear. Infrastructure providers recognize that long-term success depends on creating systems that work together seamlessly, rather than competing isolated solutions. These foundations might not generate exciting headlines, but they determine which projects succeed in bringing AI agents from concept to production. The teams solving these fundamental challenges today shape how Web3 will function tomorrow.
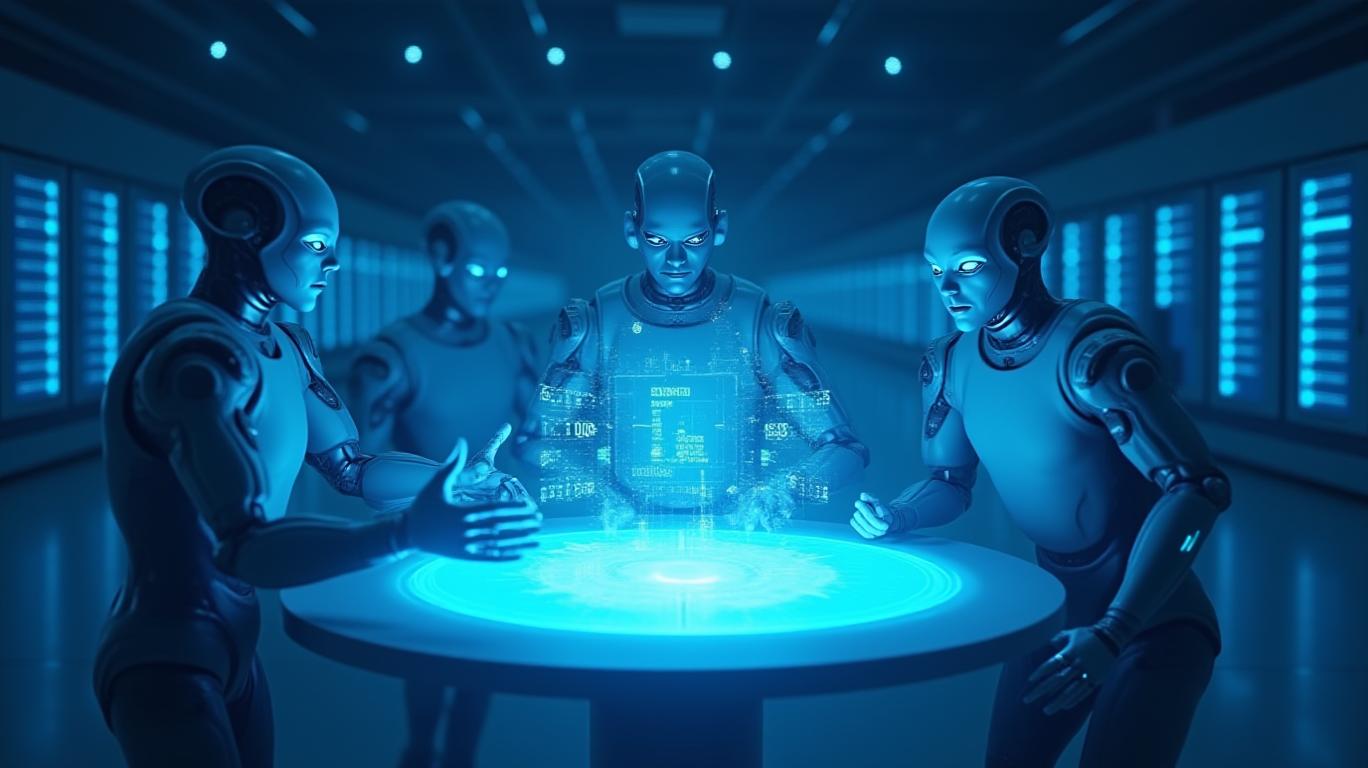